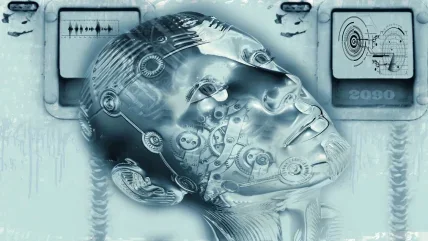
On 14 October 1962, a lone U-2 spy plane soared over western Cuba, taking 928 photographs of the island 72,500ft below. Analysing the images the next day, interpreters at the US National Photographic Interpretation Centre identified SS-4 medium-range ballistic missiles deployed outside the town of San Cristobal. US National Security Advisor McGeorge Bundy swiftly informed President John F Kennedy of the deployments the following morning.
Too often, the story of US intelligence and the ‘Cuban Missile Crisis’ is framed from this point onward. The film Thirteen Days, for example, begins with a dramatic montage of the U-2 flight proceeded by photo interrupters anxiously poring over the black and white stills.
These accounts give the impression that US surveillance operations in Cuba were immediate and effective, but the truth is somewhat different. The US actually failed to detect the secret operation to install Soviet nuclear missiles in Cuba for nearly six months, while the missiles were on the island for five weeks before being discovered.
As geospatial intelligence expert Joseph Caddell has observed, “While the intelligence community counts the missile crisis among its historic successes, it might be considered more of a near-failure.” Though it was a sizeable project – thousands of pieces of equipment and tens of thousands of personnel moving 8,000 miles, making 200 trips beginning in June 1962 – insufficient satellite coverage left the US unable to pinpoint the Soviet threat.
Only two weeks before U-2 aerial photographs confirmed the missiles, US intelligence attempted to survey the island with a spy satellite named Corona, which returned hard copy film back to earth via a parachute pod. In the end, cloud cover prevented the images from providing useful evidence. Had today’s advanced orbital satellites been available, things would surely have been different, providing a swifter, more accurate form of surveillance.
Modern surveillance
These days, such imagery is being used to measure and identify human activity around the globe, providing intelligence agencies and militaries with evidence of unlawful activity. In 2014, satellite images exposed the extent of the destruction of cultural heritage sites in northern Iraq and Syria. Only last year, satellite photographs revealed the burning of Rohingya villages in Myanmar.
As Dr Thomas Neff, head of reconnaissance and security at the German Aerospace Centre (DLR), explains, advances in satellite mapping now mean that vast swathes of Earth can be intricately mapped out in real time. The problem, however, is how to sort through this abundance of data and turn it into useful forms of intelligence.
“For a decade, we have been developing information-generation tools out of satellite-based SAR systems. Since satellite capabilities are improving, we are going to have access to more data than ever before. The only way for all this data to be used properly is to have artificial intelligence (AI) machines that can sort through it all,” Neff explains.
DLR is working with the Leibniz Computer Centre, one of Europe’s largest supercomputing groups, to evaluate satellite reports alongside global data sources such as social media networks. While the main purpose of the technology is to identify environmental patterns, such as climate change and natural disasters, Neff believes it could unlock longterm defence and security capacities.
“We are using these deep-learning methods to facilitate an automatic target-recognition system from the space-point image data,” he says. “The key is to get these machines to recognise intricate details of certain images and process that information in a usable way. This technology is very much in its infancy, but it’s not hard to see how these algorithms could enhance military surveillance in the future.”
Such methods have already been deployed to good effect. Research funded by the US Energy Department’s National Nuclear Security Administration used computer learning to co-analyse large volumes of satellite imagery and social media activity on natural disasters. By merging satellite patterns with tweets and Facebook activity from a deadly Colorado flooding in 2013, analysts believe they could glean a more accurate depiction of future events, reacting to these disasters faster and more efficiently.
“Next steps for the project include evaluating nuclear facilities in the West to identify common characteristics that may also be applicable to facilities in more isolated societies, such as North Korea,” notes a press release on the paper.
Data and detection
Given the increasing prevalence of deep fakes – machine-manipulated videos of human interactions – questions need to be asked about how this data is validated. Not only can these computergenerated videos fake human actions, but they can also trick satellites.
It has been well documented that China has experimented with generative adversarial networks to trick computers into seeing objects in landscapes or in satellite images that aren’t there. Increasingly, then, we are heading closer to a world where AI systems will be locked in a struggle for truth, with one algorithm generating erroneous content, as another is used to delegitimise it.
As Greg Allen and Taniel Chan note in the paper ‘Artificial Intelligence and National Security’, “AI can assist intelligence agencies in determining the truth, but it also makes it easier for adversaries to lie convincingly.”
Another area where AI could play an influential role is with satellites, through collision-warning and collision-avoidance systems. “Satellites currently have inbuilt sensors to detect debris,” Neff says. “The problem is that the relative velocity between the particle and the satellite means that you can’t react quickly enough on the ground to avoid this kind of threat. At the moment the only way to avoid a collision between the satellite and the debris is to use AI.”
Neff is also confident that these deep-learning capabilities can be harnessed to create advanced drone-detection systems, providing a faster way of guarding against drone attacks. However, as he warns, these devices are struggling to operate in urban environments.
68%
The amount of the world’s population living in urban areas by 2050, increasing the likelihood of urban warfare and the challenges this poses for surveillance.
UN
“The systems that we have are not working well in cities because it’s far harder to see anything,” he says, “So you can only see a drone from 50m away, because it’s behind a house or a wall. And, given that more fighting is going to take place in urban areas, you need to find a solution for that.” This is a pressing problem, as a UN report estimates that 68% of the world’s population will be living in urban areas by 2050.
There is another issue here, however, given that this same image analysis technology is being used to enhance drone surveillance capabilities. As retired US Navy Commander Ted Johnson and Air Force General Charles F Wald have said in a joint statement, “The use of unmanned aircraft systems for surveillance and intelligence may turn out to be a more revolutionary development than the drone strikes themselves.”
Under review
Such developments will only work if military operators can analyse huge samples of collected video. A dull task, given that around 60% of drone video holds little to no value to military operations.
To solve this quandary, the Pentagon has been partnering with Google to develop autonomous, computer-enabled analysis techniques. In March 2018, it was announced that the US Government was working with the technology giant to help air force analysts sort through thousands of hours of drone video to choose more clearly defined targets on the battlefield. The initiative, code named ‘Project Maven’, uses machine-learning capabilities to distinguish between buildings, trees and other materials, and locate targets autonomously.
Senior Google executives have since renounced the programme, with more than 3,000 Google employees signing an open letter to Google CEO Sundar Pichai, declaring that “Google should not be in the business of war”. However, as technology journalist Kate Conger has observed, the company has not explicitly ruled out future collaborations.
As a result of military actions in Iraq, Syria, Afghanistan and Yemen, the Pentagon estimated that nearly 500 civilians were killed as a result of military airstrikes in 2017, with approximately 169 civilians badly injured. In the future, AI could be used to reduce such collateral damage, avoiding accidental casualties caused by less precise forms of weaponry.
Nevertheless, the ethical implications of the project are disturbing, given that even a small task such as combing through footage can have a huge influence on battlefield decision-making.
As political scientist Michael C Horowitz explains – in a paper entitled ‘The promise and peril of military applications of artificial intelligence’ – the very nature of deep machine learning, “which means a machine determining the best action and taking it, makes it hard to predict the behaviour of AI systems”.
This is famously known as the ‘black box’ problem of deep learning. Even if an AI system makes a seemingly correct decision, its motives are buried in layers of computations that are both mind numbingly complex and, at times, completely inexplicable.
For Neff, this lack of transparency is currently the single biggest problem for incorporating AI systems into military operations. “If you do anything with AI, there is an aspect to algorithmic decisionmaking that nobody understands. In military operations things have to be transparent, but, particularly with more complex AI systems, there is no clear way of seeing why a particular decision has been made,” he explains.
This grey area was famously exposed in a match of Go – the ancient board game – between world champion Lee Sedol and Google’s DeepMind playing machine, named AlphaGo. The second game included a moment when AlphaGo made a move so unusual that Sedol left the room for 15 minutes to consider what had just happened. It was only many moves later that onlookers realised the brilliance of the manoeuvre; a move so surprising that it had overturned hundreds of years of received wisdom.
While the genius of such a complex decisionmaking process is tantalisingly evident to Go players and chess champions, for militaries – which run on precedent and trust – it is less appealing. If an AI system classifies an image or destroys a drone, but cannot explain why that decision was taken, it is essentially a rogue agent.
Due to its unpredictable and opaque decisionmaking, then, AI technology currently poses risks that might be too great for military programmes to take. Inevitably, it poses difficult questions about the role of trust and accountability in military operations.
But given the breadth of the technology and the myriad ways it is being used in our everyday lives, it seems more likely that the age of AI is set to shape the future of militaries around the world.